Regenerative AI is an emerging concept that expands upon the traditional definitions of artificial intelligence, emphasizing sustainability, positive environmental impact, and the integration of ecological principles into AI development and application. Here's a breakdown of its core ideas:
Key Features of Regenerative AI:
Environmentally Conscious AI Development:
Prioritizes the use of energy-efficient algorithms and carbon-neutral computation.
Embeds nature protection and regeneration into the development of AI Agents on Web3
Focuses on reducing the ecological footprint of AI models, especially during training, which is typically energy-intensive.
It makes emphasis on Decentralized AI storage and hosting
Applications in Environmental Restoration:
Designs AI systems to support reforestation, carbon sequestration, biodiversity mapping, and other ecological restoration projects.
Uses AI to monitor ecosystems and predict environmental changes, aiding in sustainability planning.
Circular Data Economy:
Encourages the recycling and repurposing of data for AI training rather than creating excessive, resource-intensive new datasets.
Promotes data-sharing frameworks that benefit multiple stakeholders in a sustainable and equitable manner.
Ethical Decision-Making:
Integrates ethical principles that align with regenerative philosophies, ensuring AI systems respect both human and non-human life.
Strives to minimize harm to ecosystems and communities, considering the broader implications of AI interventions.
Support for Regenerative Economies:
Powers tools for decentralized finance (DeFi), tokenized land, carbon credits, and biodiversity credits, driving investments into projects with real-world ecological benefits.
Enhances local, community-driven initiatives through automated management and predictive modeling.
Integration with Regenerative Finance (ReFi):
Collaborates with regenerative finance principles to align AI models with sustainable economic goals.
Leverages blockchain and decentralized platforms to track, verify, and fund regenerative projects transparently.
Interdisciplinary Approach:
Combines knowledge from AI, biology, environmental science, and economics to create holistic systems.
Encourages collaboration between scientists, engineers, and ecologists to ensure alignment with natural processes.
Example Applications:
Smart Agriculture: AI tools that improve soil health, optimize water use, and enhance crop yield sustainably.
Energy Optimization: Predictive systems for managing renewable energy grids and reducing energy waste.
AI-Driven Conservation: Monitoring endangered species and preserving habitats using AI-powered drones and sensors.
Climate Action Platforms: AI systems to calculate and reduce individual or organizational carbon footprints.
Conclusion:
Regenerative AI isn’t just about creating smarter machines; it’s about ensuring those machines actively contribute to the health and vitality of the planet. It is closely tied to principles of sustainability, equity, and innovation, aiming to harmonize technology with the natural world rather than exploiting it.
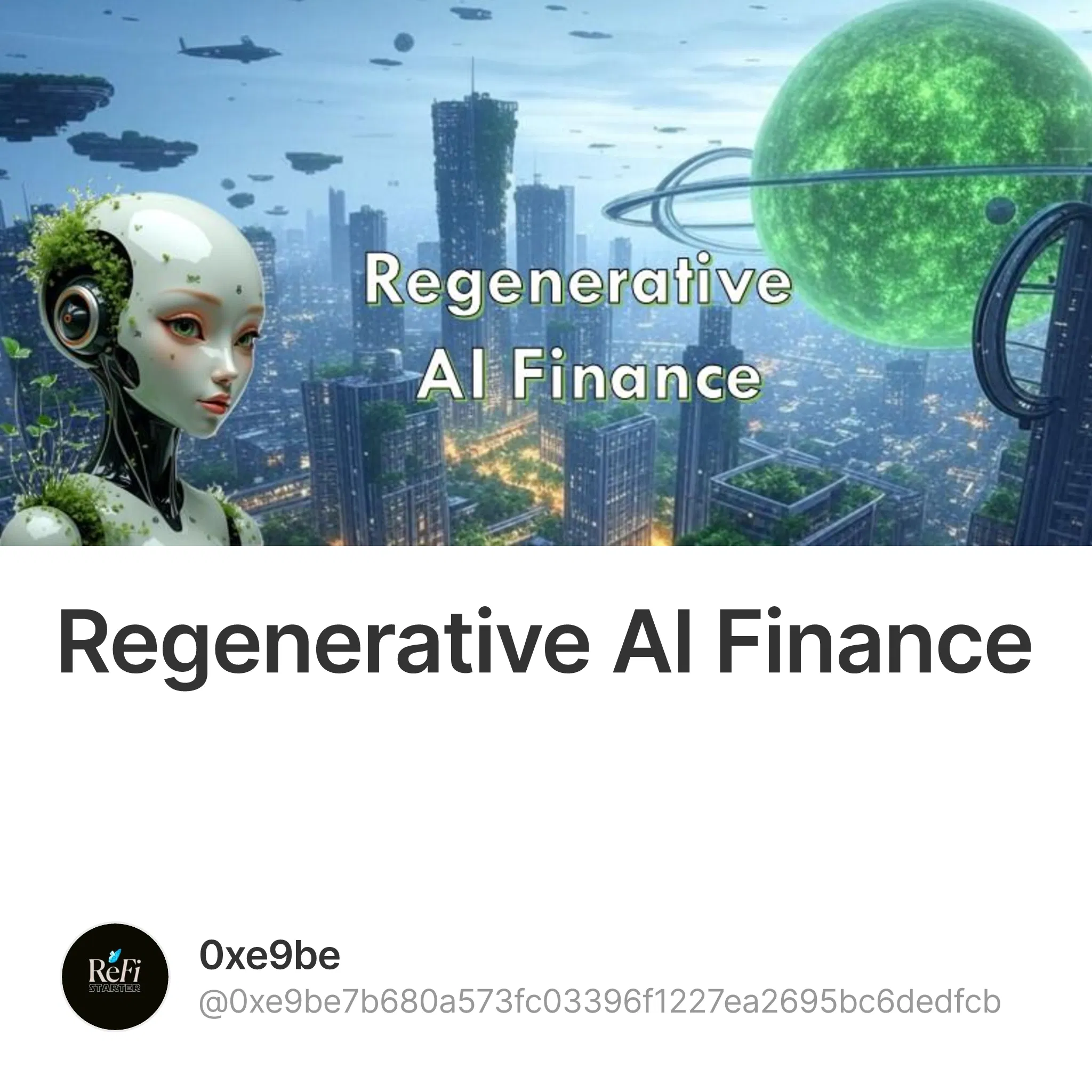
