Like any other process, AI also produces a carbon footprint, which can be significant in some instances and inaccurately measured in others.
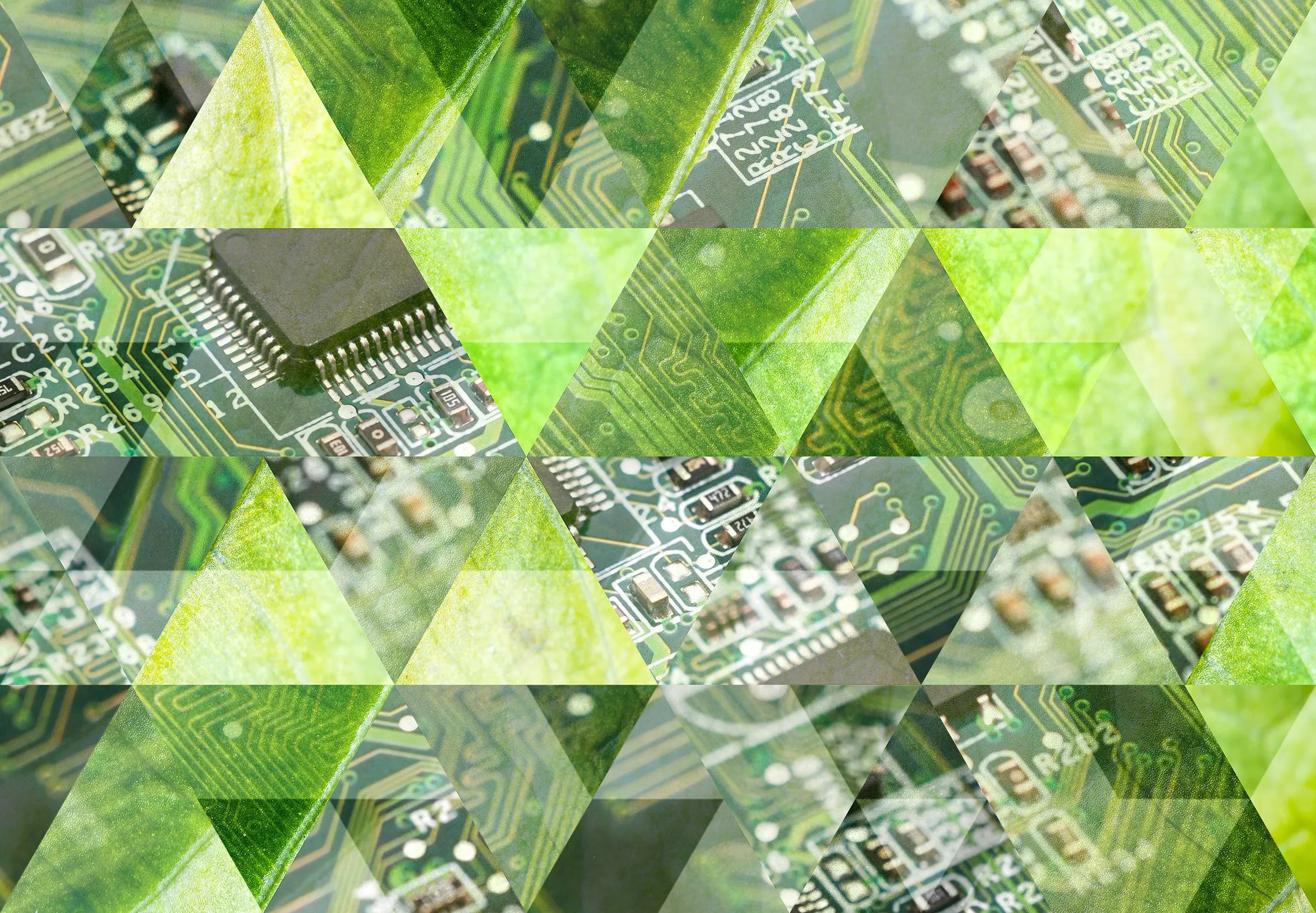
Research and testing revealed that the energy used to power GPUs for training the smallest models resulted in carbon emissions equivalent to charging a smartphone. The largest model, which contained six billion parameters, emitted nearly as much carbon as the annual energy consumption of a U.S. household when trained to just 13 percent completion. In contrast, deployed models like OpenAI's GPT-3 have over 100 billion parameters.
The implications of these findings are profound, especially when considering the exponential growth in AI model sizes and their deployment across various industries. As models continue to scale, so too does their energy consumption and corresponding carbon footprint. This situation underscores the critical need for more efficient algorithms, renewable energy sources, and innovative cooling technologies to mitigate environmental impact. Furthermore, the disparity between the carbon emissions of smaller and larger models highlights the importance of conscientious development practices and the consideration of ecological costs in AI research and application.
Research and verification in this new area is the need of the hour for the safety and better future of our future generations and our planet.
It’s essential for the AI community to prioritize sustainable practices to reduce the environmental impact of advanced AI models.
You can read more about this topic here:
AI in the 2020s Must Get Greener—and Here’s How - IEEE Spectrum
AI’s Carbon Footprint Problem (stanford.edu)
You can follow me on other platforms as well:
References

