Everyone’s asking what AI tools you’re using these days. New ones are dropping every week and it's hard to keep up. I do my best to experiment with different ones and am constantly swapping things in and out. But I’ve begun to notice a meaningful difference in which tools stick and which end up collecting dust.
The tools that stick for me are those that have a unique and near frictionless mechanism of data capture natively embedded in their UX. I’ve started to call this a “data spring.”
Here’s what I mean. Last year, meeting bots began popping up in our zooms almost overnight. Having an AI transcribe and summarize notes felt big. I tried out a bunch of different meeting bots but found it a nightmare to get the right one to show up at the right time. Sometimes it wouldn’t show up to the meeting at all or, even more bizarrely, it would show up late.
Then I started using granola. It felt like magic to click a button and have the desktop app transcribe any meeting. It was dead simple, and I could steer the summary by jotting down notes of my own too. Since then, I've used the app almost daily.
That button is a data spring. Think of it like trying to install a tap in a maple tree. You’re scraping away at the bark, drilling random holes, thinking of just chopping down the whole damn tree. Then, almost by chance, you find that one ideal spot on the tree, make a small incision, and you get maple syrup. That’s what a good data spring does: it’s the singular magic point where valuable data begins to flow effortlessly, turning a laborious process into a deceptively simple one.
Lots of different data springs come to mind. Screenshotting your phone. Whipping out shazam at a party. Crypto-incentivized web scraping. Springs can be more multi-staged too. I think of Evvy, a women’s health startup, that’s amassed a rich database of vaginal microbiome data by making an at-home test for women who felt dismissed by institutional healthcare.
Future springs are hard to imagine but inevitable. As multimodal models get better and cheaper, there will be more weird and wacky ways to mine new kinds of data and make it useful.
You can’t build a venture-scale company on a data spring or data asset alone. Foundation models are becoming increasingly versatile. Domain-specific models with proprietary datasets could be outperformed one day by advancing foundation models. And aside from that scenario, apps will still have to deliver ROI for the data they receive.
When used thoughtfully though, the right data spring will do more than create a decent head start. It can give the product a kind of physics that fundamentally propels it forward into the hands of users. As AI continues to evolve, identifying and leveraging these critical capture points will become a key differentiator in the success of future AI tools.
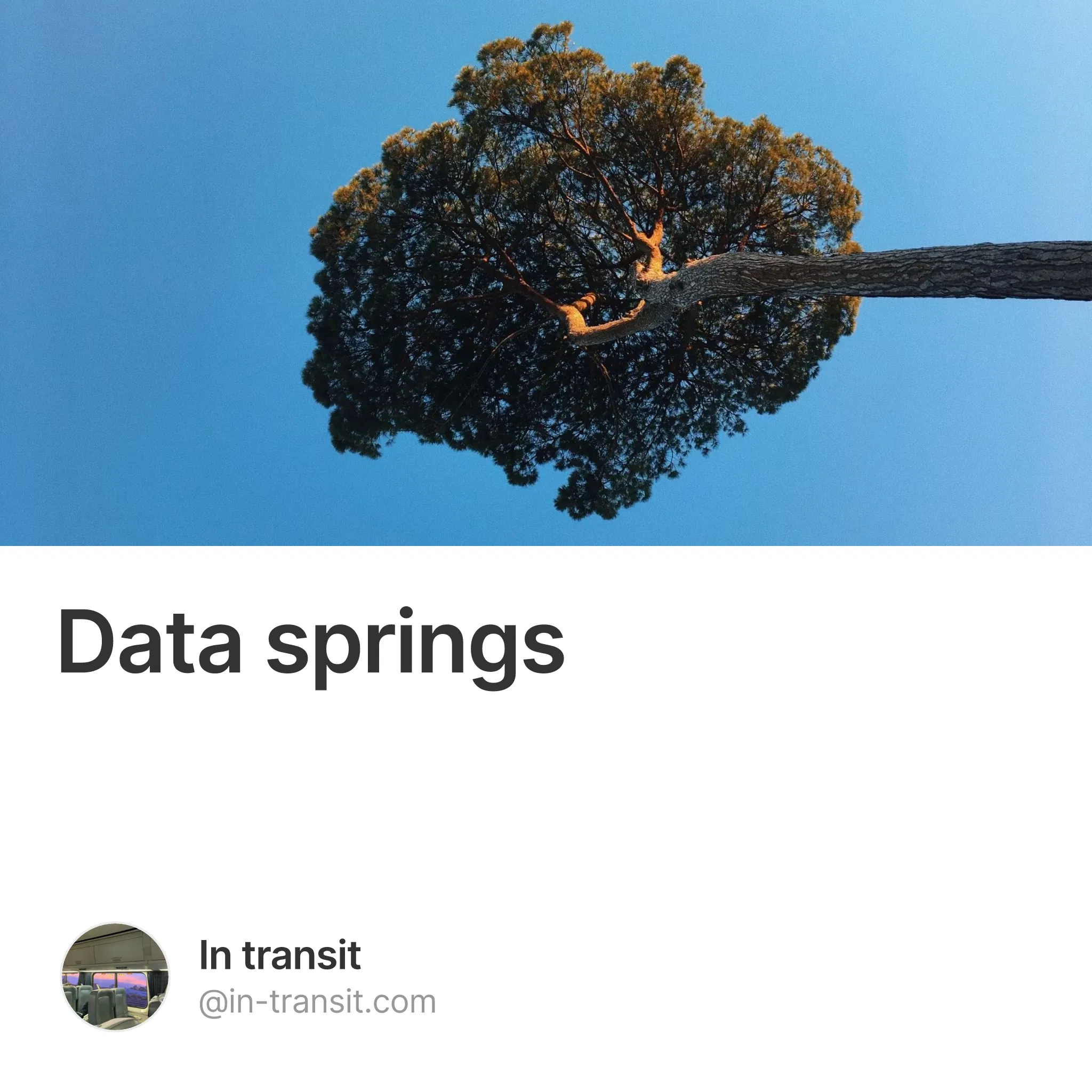
