Good Morning!
Delivering interesting content every single week on Web3, Security, Crypto, NFTs, Design & AI.
It's FREE, Takes less than 5-minutes to read, and you are guaranteed to learn something.
Subscribe to get valuable Web3 News, Useful Resources and Insights every week to your Inbox!
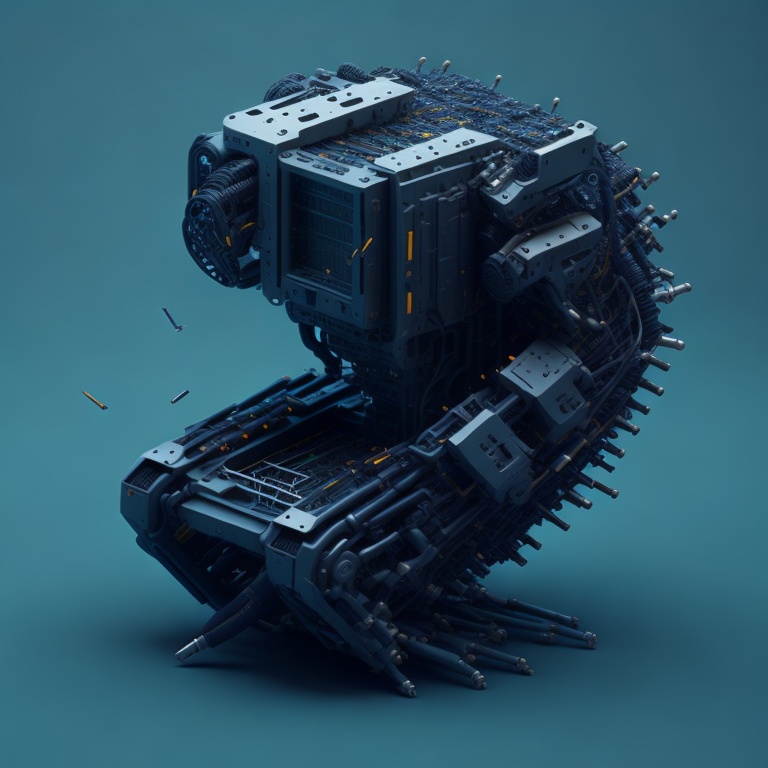
Zero-knowledge machine learning (ZKML) is an emerging field that addresses the challenges of privacy and confidentiality in machine learning. In traditional machine learning, data is typically collected and analyzed by a central authority, which presents significant risks in terms of data breaches, privacy violations, and misuse of sensitive information. ZKML, on the other hand, enables data analysis without revealing the raw data or sensitive information.
The concept of zero-knowledge proof (ZKP) serves as a fundamental building block of ZKML. A zero-knowledge proof is a cryptographic protocol that allows one party (the prover) to demonstrate to another party (the verifier) that they know a specific piece of information without revealing the information itself. In the context of machine learning, a zero-knowledge proof allows a machine learning model to prove its accuracy without revealing the underlying data.
ZKML can be applied to various domains, such as healthcare, finance, and social media. For instance, ZKML can be used to develop machine learning models for disease prediction without compromising patient privacy. ZKML can also be applied in financial institutions to predict credit scores without exposing sensitive information about individuals.
One of the challenges in ZKML is maintaining the balance between privacy and accuracy. In traditional machine learning, models are trained on large datasets, which enables the model to make accurate predictions. However, in ZKML, the data remains private, and therefore, it is challenging to train a model on a large dataset. As a result, the accuracy of the model may be compromised, and it may require more iterations to achieve reasonable performance.
There are several approaches to ZKML, including homomorphic encryption, secure multi-party computation, and differential privacy. Homomorphic encryption is a technique that enables computation on encrypted data without decrypting it, while secure multi-party computation enables multiple parties to compute a function while keeping their inputs private. Differential privacy, on the other hand, adds noise to the data to preserve privacy while maintaining the accuracy of the machine learning model.
Another challenge in ZKML is the complexity of the cryptographic protocols. ZKP, homomorphic encryption, and secure multi-party computation all involve complex mathematical algorithms and computational overheads, which can make ZKML models computationally expensive. However, recent advances in cryptography and hardware have enabled the development of efficient ZKML models that can handle large datasets.
ZKML also raises ethical concerns, such as the potential misuse of the technology for malicious purposes. For instance, ZKML can be used to develop models that predict sensitive information, such as political affiliations, sexual orientation, or religious beliefs, which can be used to discriminate against individuals or groups. Therefore, it is essential to develop ethical guidelines and regulations to ensure the responsible use of ZKML.
In conclusion, ZKML is an exciting and rapidly evolving field that has the potential to transform machine learning while addressing the challenges of privacy and confidentiality. ZKML provides a novel approach to data analysis that enables accurate predictions without compromising sensitive information. However, ZKML also presents several challenges, including maintaining the balance between privacy and accuracy, complexity of cryptographic protocols, and ethical concerns. Further research is required to develop efficient and secure ZKML models that can handle large datasets while ensuring the responsible use of the technology.
I hope this was helpful!
Thank you for reading!
If you're interested in following along, feel free to subscribe!
Let’s bust some more in next article.
If you want more, be sure to
FOLLOW ME
